Speakers

Julian McAuley / UC San Diego
In this talk, I'll present several case studies demonstrating the risks involved in deploying personalized machine learning algorithms, especially recommender systems. Examples range from problems of mainstream interest (such as filter bubbles and 'extremification'), to more subtle forms of unfairness, such as the loss of utility felt by users whose demographics are poorly represented by marketing content. I'll also discuss various strategies to mitigate these undesired effects, by adapting ideas from 'fair ML' to recommendation scenarios.
Speaker Bio: Julian McAuley is a Professor at UC San Diego, where he works on applications of machine learning to problems involving personalization, and teaches classes on personalized recommendation. He likes bicycling and baroque keyboard.

David Carmel / Amazon Sciences
In this talk I'll discuss the fundamentals of personalized shopping in
eCommerce using voice-based AI assistants like Amazon's Alexa. I'll
cover some of the recent works done in our lab on personalized
shopping experience with Alexa, with the focus on product search and
product question answering.
Speaker Bio: David is a Principal Applied Scientist at Amazon, Israel and an ACM
Distinguished Engineer. His current research is focused on improving
product search and product question answering through Amazon’s Alexa.
David has published more than 150 papers in IR and Web journals and
conferences. He served as a general co-chair for WSDM 2021, a
technical program co-chair for CIKM 2019, and an area chair and SPC in
many IR and Web conferences. David earned his PhD in Computer Science
from the Technion, Israel Institute of Technology in 1997.
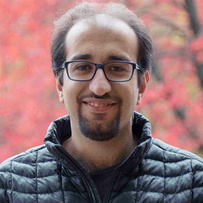
Hamed Zamani / UMass Amherst
Despite the somewhat different techniques used in developing search
engines and recommender systems, they generally follow a common goal:
helping people to get the information they need at the right time.
Therefore, search and recommendation models can potentially benefit
from each other. The recent advances in neural network technologies
make them effective and easily extendable to various tasks, including
retrieval and recommendation. This raises the possibility of jointly
modeling and optimizing search ranking and recommendation algorithms,
with potential benefits to both. In this talk, I will highlight
challenges we face in joint modeling of search and recommendation as
well as the opportunities it introduces, such as improving model
generalization, interpretability, and explainability. I will further
discuss the applications of joint search and recommendation models to
conversational AI systems.
Speaker Bio: Hamed Zamani is an Assistant Professor in the Manning College of
Information and Computer Sciences (CICS) at the University of
Massachusetts Amherst (UMass), where he also serves as the Associate
Director of the Center for Intelligent Information Retrieval (CIIR).
Prior to UMass, he was a Researcher at Microsoft. In 2019, he received
his Ph.D. from UMass under supervision of W. Bruce Croft and received
the CICS Outstanding Dissertation Award. His research focuses on
developing and evaluating statistical and machine learning models with
application to (interactive) information access systems including
search engines, recommender systems, and question answering. He is an
active member of the information retrieval community and has published
over 70 peer-reviewed articles. He is mostly known for his work on
neural information retrieval and conversational search. His papers
have received awards from ICTIR 2019 and CIKM 2020. Most recently, his
research group has been selected for the Alexa Prize Challenge 2021.
He has organized multiple workshops at SIGIR, WSDM, and RecSys and is
currently serving as the Program Committee Co-Chair for SIGIR 2022 -
Short Paper Track.